Researcher Earns Grant to Develop New Social Behavior Models
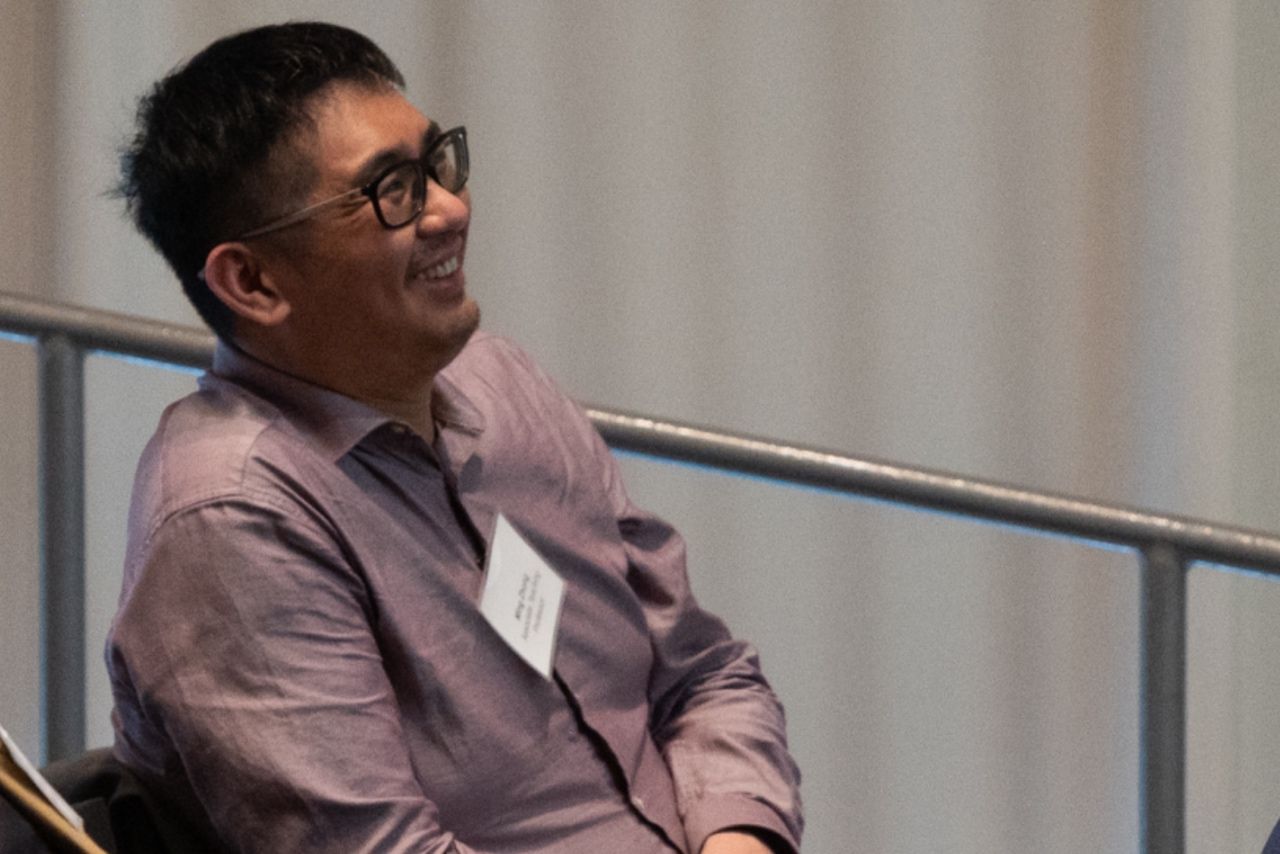
Mathematical models have been used to simulate and predict emergent behaviors in nature such as birds flocking, bees swarming, and fish milling in a school, but an Illinois Institute of Technology researcher is developing a new model to predict social emergent behaviors.
Ming Zhong, assistant professor of applied mathematics, has earned a Ralph E. Powe Junior Faculty Enhancement Award from Oak Ridge Associated Universities to develop two distinct data-driven modeling methods that are designed for simulating and predicting emergent behaviors from the complex systems. The models will be designed to apply to social issues such as market economy, opinion formation, and criminal justice. He is one of 37 recipients who were chosen from 174 applicants.
“That we can get recognition by ORAU to embark on a research project that might actually affect civic policies and have real impact on the society is exciting,” Zhong says. “We also look for more and future collaborations with national labs, companies, and other civil government branches to understand our model better.”
The focus of the research is to understand emergent behaviors in the modeling of social issues, such as the formation of the polarized American political landscape. The objective is to offer mathematical insights into how modeling such behaviors can not only enhance the understanding of daily American life, but also contribute to improving living standards and the efficiency of civil governance.
“Clustering, flocking, and swarming are emerging patterns that are generated by locally interacting agents,” Zhong says. “These agents can be intelligent agents, such as humans. Social behaviors can demonstrate certain interesting patterns, for example, traffic flow, market economics, group think, or herd behavior. Consensus of political opinions can be thought of as a form of clustering.”
Zhong says the research will result in new data-driven modeling techniques by a variational inverse problem to derive a more realistic model from using actual data obtained from the Chicago public database.
“There is more randomness in intelligent agent behaviors, such as free wills, than in particles or other non-intelligent agents,” Zhong says. “To model these behaviors more accurately, one has to understand the underlying causes of these patterns, such as how the agents would influence and interact with each other.”
Zhong says the biggest challenge the project presents is cleaning the real data sets to be used in the models.
“Real data sets might have a lot of noise, presents gaps in the models, and other various factors that might prevent us to predict well,” he says.